In the last few weeks we introduced a number of features to improve your experience with our models. We hope they will make it easier for you to test, develop, and productionize the solutions built on top of Luminous. In this changelog we want to inform you about the following changes:
You can directly access the tokenizer
we use in our models. This allows you to count the number of tokens in your prompt more accurately.
Previously, you had to use separate methods for different image sources (URLs or local file paths). With our new method Image.from_image_source
, you can just pass in the URL, local file path, or bytes array, and we will do the heavy lifting in the background.
You can use the completion_bias_inclusion
parameter to limit the output tokens to a set of allowed keywords.
We added a minimum tokens
parameter. It guarantees that the answer will always have at least k
tokens and not end too soon.
Sometimes our models tend to repeat the input prompt or previously generated words in the completion. We are introducing multiple new parameters to address this problem.
We added the echo
parameter. We can return not only the completion but also the prompt, which might be useful for benchmarking.
Embeddings can now come in normalized form.
New Python Client Features
Local Tokenizer
You can now directly access the tokenizer
we use in our models. This allows you to count the number of tokens in your prompt more accurately.
import os
from aleph_alpha_client import Client
client = Client(token=os.getenv("AA_TOKEN"))
tokenizer = client.tokenizer("luminous-supreme")
text = "Friends, Romans, countrymen, lend me your ears;"
tokens = tokenizer.encode(text)
and_back_to_text = tokenizer.decode(tokens.ids)
print("Tokens:", tokens.ids)
print("Back to text from ids:", and_back_to_text)
Tokens: [37634, 15, 51399, 15, 6326, 645, 15, 75938, 489, 867, 47317, 30]
Back to text from ids: Friends, Romans, countrymen, lend me your ears;
Unified method to load images
Previously, you had to use separate methods for different image source (URLs or local file paths). With our new method Image.from_image_source
, you can just pass in the URL, local file path or bytes array and we will do the heavy lifting in the background.
import os
from aleph_alpha_client import Client, Prompt, CompletionRequest, Image
client = Client(token=os.getenv("AA_TOKEN"))
image_source = "https://docs.aleph-alpha.com/assets/images/starlight_lake-296ab6aa851c37de66e6b5afe046f12e.jpg"
image = Image.from_image_source(image_source=image_source)
prompt = Prompt(
items=[
image,
"Q: What is known about the structure in the upper part of this picture?\nA:",
]
)
params = {
"prompt": prompt,
"maximum_tokens": 16,
"stop_sequences": [".", ",", "?", "!"],
}
request = CompletionRequest(**params)
response = client.complete(request=request, model="luminous-extended")
completion = response.completions[0].completion
print(completion)
New Completion Parameters
Limit the model outputs to a set of allowed words
If you want to limit the model's output to a pre-defined set of words, use the completion_bias_inclusion
parameter. One possible use case for this is classification, where you want to limit the model’s output to a set of pre-defined classes. The most basic use case would be a simple binary classification (e.g., "Yes" and "No"), but it can be extended to more advanced classification problems like the following:
import os
from typing import List
from aleph_alpha_client import Client, Prompt, CompletionRequest
client = Client(token=os.getenv("AA_TOKEN"))
def classify(prompt_text: str, key: str, values: List[str]) -> str:
prompt = Prompt.from_text(prompt_text)
completion_bias_request = CompletionRequest(
prompt=prompt,
maximum_tokens=20,
stop_sequences=["\n"],
completion_bias_inclusion=values,
)
completion_bias_result = client.complete(
completion_bias_request, model="luminous-extended"
)
return completion_bias_result.completions[0].completion.strip()
prompt_text = """Extract the correct information from the following text:
Text: I was running late for my meeting in the bavarian capital and is was really hot that day, especially, as the leaves were already starting to fall. I never thought the year 2022 would be this crazy.
{key}:"""
key = "temperature"
text_classification_inclusion_bias = classify(
prompt_text=prompt_text, key=key, values=["low", "medium", "high"]
)
text_classification_standard = classify(prompt_text=prompt_text, key=key, values=None)
print(f"Inclusion bias {key} classification: {text_classification_inclusion_bias}")
print(
f"{key} classification without the inclusion bias: {text_classification_standard}"
)
print()
key = "Venue"
text_classification_inclusion_bias = classify(
prompt_text=prompt_text, key=key, values=["Venice", "Munich", "Stockholm"]
)
text_classification_standard = classify(prompt_text=prompt_text, key=key, values=None)
print(f"Inclusion bias {key} classification: {text_classification_inclusion_bias}")
print(
f"{key} classification without the inclusion bias: {text_classification_standard}"
)
print()
key = "Season"
text_classification_inclusion_bias = classify(
prompt_text=prompt_text, key=key, values=["Spring", "Summer", "Autumn", "Winter"]
)
text_classification_standard = classify(prompt_text=prompt_text, key=key, values=None)
print(f"Inclusion bias {key} classification: {text_classification_inclusion_bias}")
print(
f"{key} classification without the inclusion bias: {text_classification_standard}"
)
print()
key = "Year"
text_classification_inclusion_bias = classify(
prompt_text=prompt_text, key=key, values=["2019", "2020", "2021", "2022", "2023"]
)
text_classification_standard = classify(prompt_text=prompt_text, key=key, values=None)
print(f"Inclusion bias {key} classification: {text_classification_inclusion_bias}")
print(
f"{key} classification without the inclusion bias: {text_classification_standard}"
)
If we limit the model only to the allowed set of classes, we get nice results, providing some classification for a given text. In this example, if we let the model use any tokens, it starts with an \n
token and the model just stops, without producing any useful output.
Inclusion bias temperature classification: high
temperature classification without the inclusion bias:
Inclusion bias Venue classification: Munich
Venue classification without the inclusion bias:
Inclusion bias Season classification: Autumn
Season classification without the inclusion bias:
Inclusion bias Year classification: 2022
Year classification without the inclusion bias:
Minimum tokens
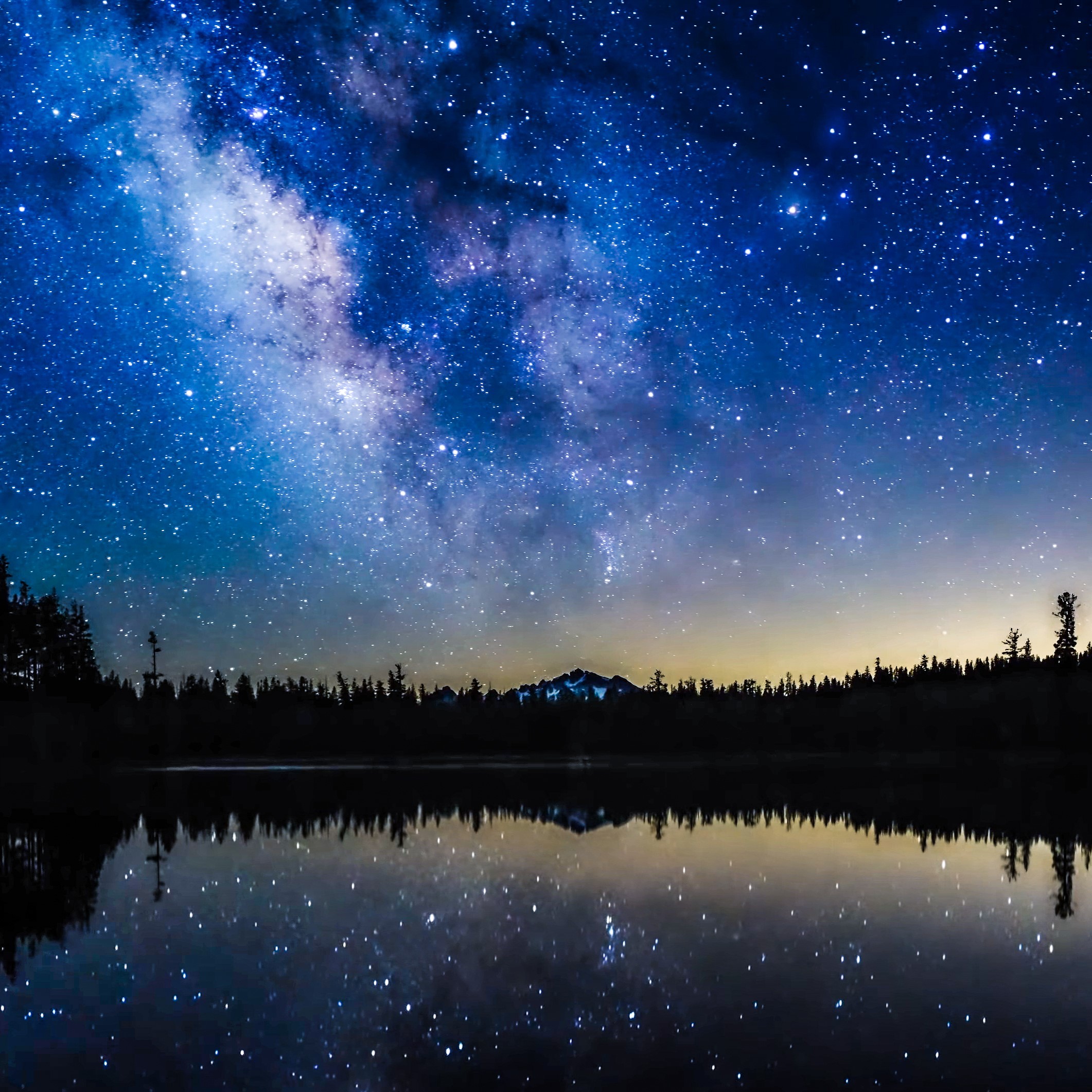
We added a minimum_tokens
parameter to the completion API. Now we can guarantee that the answer will always have at least k
tokens and not end too early. This could come in handy if you want to incentivize the model to provide more elaborate answers and not finish the completion prematurely. We hope to improve your experience with multimodal inputs and MAGMA to generate more elaborate completions. In the following example, we show you how this impacts the model’s behaviour and produces a better completion.
import os
from aleph_alpha_client import Client, Prompt, CompletionRequest, Image
client = Client(token=os.getenv("AA_TOKEN"))
image_source = "https://docs.aleph-alpha.com/assets/images/starlight_lake-296ab6aa851c37de66e6b5afe046f12e.jpg"
image = Image.from_url(url=image_source)
prompt = Prompt(
items=[
image,
"An image description focusing on the features and amenities:",
]
)
no_minimum_tokens_params = {
"prompt": prompt,
}
no_minimum_tokens_request = CompletionRequest(**no_minimum_tokens_params)
no_minimum_tokens_response = client.complete(request=no_minimum_tokens_request, model="luminous-extended")
no_minimum_tokens_completion = no_minimum_tokens_response.completions[0].completion
print("Completion with minimum_tokens not set: ", no_minimum_tokens_completion)
minimum_tokens_params = {
"prompt": prompt,
"minimum_tokens": 16,
"stop_sequences": ["."],
}
minimum_tokens_request = CompletionRequest(**minimum_tokens_params)
minimum_tokens_response = client.complete(request=minimum_tokens_request, model="luminous-extended")
minimum_tokens_completion = minimum_tokens_response.completions[0].completion.strip()
print("Completion with minimum_tokens set: ", minimum_tokens_completion)
When not using the minimum_tokens
parameter, the model starts with an End-Of-Text (EOT
) token and produces an empty completion. With the minimum_tokens
in place, we get a proper description of the image.
Completion with minimum_tokens not set:
Completion with minimum_tokens set: The Milky Way over a lake in the mountains
Preventing repetitions
Sometimes our models tend to repeat the input prompt or previously generated words in the completion. With a combination of multiple new parameters (repetition_penalties_include_prompt
, repetition_penalties_include_completion
, sequence_penalty_min_length
, use_multiplicative_sequence_penalty
) you can prevent such repetitions. To learn more about them, check out the API documentation.
To use the feature, just add the parameters to your request and get rid of unwanted repetitions. An example use case for this feature is summarization.
import os
from aleph_alpha_client import Client, Prompt, CompletionRequest
client = Client(token=os.getenv("AA_TOKEN"))
prompt_text = """Summarize each text.
###
Text: The text describes coffee, a brown to black, psychoactive, diuretic, and caffeinated beverage made from roasted and ground coffee beans and hot water. Its degree of roasting and grinding varies depending on the preparation method. The term "coffee beans" does not mean that the coffee is still unground, but refers to the purity of the product and distinguishes it from substitutes made from ingredients such as chicory, malted barley, and others. Coffee is a beverage that people enjoy and contains the vitamin niacin. The name "coffee" comes from the Arabic word "qahwa," which means "stimulating drink."
Summary: Coffee is a psychoactive and diuretic beverage made from roasted and ground coffee beans that is enjoyed for its caffeine content and prepared with varying degrees of roasting and grinding.
###
Text: Marc Antony was a Roman politician and military leader who played an important role in the transformation of the Roman Republic into the autocratic Roman Empire. He was a follower and relative of Julius Caesar and served as one of his generals during the conquest of Gaul and the Civil War. While Caesar eliminated his political opponents, Antony was appointed governor of Italy. After Caesar's assassination, Antony joined forces with Marcus Aemilius Lepidus and Octavian, Caesar's grandchild and adopted son, to form the Second Triumvirate, a three-man dictatorship. The Triumvirate defeated Caesar's murderous liberators at the Battle of Philippi and divided the republic's administration between them. Antony was given control of Rome's eastern provinces, including Egypt, ruled by Cleopatra VII Philopator, and was charged with leading Rome's war against Parthia.
Summary:"""
no_repetition_management_params = {
"prompt": Prompt.from_text(prompt_text),
"stop_sequences": ["\n", "###"]
}
no_repetition_management_request = CompletionRequest(**no_repetition_management_params)
no_repetition_management_response = client.complete(request=no_repetition_management_request, model="luminous-extended")
no_repetition_management_response = no_repetition_management_response.completions[0].completion
print("No sequence penalties:")
print(no_repetition_management_response.strip())
print()
print("Repetition management:")
repetition_management_params = {
"prompt": Prompt.from_text(prompt_text),
"repetition_penalties_include_prompt": True,
"repetition_penalties_include_completion": True,
"sequence_penalty":.7,
"stop_sequences": ["\n", "###"]
}
repetition_management_request = CompletionRequest(**repetition_management_params)
repetition_management_response = client.complete(request=repetition_management_request, model="luminous-extended")
repetition_management_response = repetition_management_response.completions[0].completion
print("Sequence penalties:")
print(repetition_management_response.strip())
When the sequence penalty is not applied, models tend to repeat the first sentence of the text they are supposed to summarize ("Marc Antony was a Roman politician …"). When applying the sequence penalty parameter, this is no longer the case. The output that we get is better, more creative, and, most importantly, isn’t just a repetition of the first sentence.
No sequence penalties:
Marc Antony was a Roman politician and military leader who played an important role in the transformation of the Roman Republic into the autocratic Roman Empire.
Sequence penalties:
Marc Anthony was an important Roman politician who served as a general under Julius Caesar
Echo for completions
We added the echo
parameter. Setting this parameter will not only return the completion but also the prompt. If you run benchmarks on our models, the parameter allows you to get log probs for input and output tokens. This makes us compatible with other APIs that do not provide an evaluate endpoint. To use the functionality, set the echo parameter to True
.
import os
from aleph_alpha_client import Client, Prompt, CompletionRequest
client = Client(token=os.getenv("AA_TOKEN"))
prompt_text = "If it were so, it was a grievous fault,\nAnd"
params = {
"prompt": Prompt.from_text(prompt_text),
"maximum_tokens": 16,
"stop_sequences": ["."],
"echo": True
}
request = CompletionRequest(**params)
response = client.complete(request=request, model="luminous-extended")
completion_with_echoed_prompt= response.completions[0].completion
print(completion_with_echoed_prompt)
If it were so, it was a grievous fault,
And grievously hath Caesar answer’d it
Embeddings
Embeddings normalization
We now provide you with the option to normalize our (semantic) embeddings, meaning that the vector norm of the result embedding is 1.0. This can be useful in applications where you need to calculate the cosine similarity. To use it, just set the normalize
parameter to True
.
import os
from aleph_alpha_client import Client, Prompt, SemanticRepresentation, SemanticEmbeddingRequest
import numpy as np
text = "I come to bury Caesar, not to praise him."
client = Client(token=os.getenv("AA_TOKEN"))
non_normalized_params = {
"prompt": Prompt.from_text(text),
"representation": SemanticRepresentation.Symmetric,
"compress_to_size": 128,
"normalize": False
}
non_normalized_request = SemanticEmbeddingRequest(**non_normalized_params)
non_normalized_response = client.semantic_embed(request=non_normalized_request, model="luminous-base")
non_normalized_response.embedding
normalized_params = {
"prompt": Prompt.from_text(text),
"representation": SemanticRepresentation.Symmetric,
"compress_to_size": 128,
"normalize": True
}
normalized_request = SemanticEmbeddingRequest(**normalized_params)
normalized_response = client.semantic_embed(request=normalized_request, model="luminous-base")
normalized_response.embedding
print("Normalized vector:", normalized_response.embedding[:10])
print(f"Non-normalized embedding sum: {np.linalg.norm(non_normalized_response.embedding):.2f}")
print(f"Normalized embedding sum: {np.linalg.norm(normalized_response.embedding):.2f}")
Normalized vector: [0.08886719, -0.018188477, -0.06591797, 0.014587402, 0.07421875, 0.064941406, 0.13183594, 0.079589844, 0.10986328, -0.12158203]
Non-normalized embedding sum: 27.81
Normalized embedding sum: 1.00